Procurement Analytics Demystified
Procurement analytics is transforming the way businesses handle their S-2-P processes by turning raw data into actionable insights.
This comprehensive guide to procurement analytics demystifies the complexities of data-driven procurement, offering step-by-step insights into how to harness the power of data to improve sourcing, supplier relationships, and cost management.
Learn how procurement analytics can help you gain a competitive edge, streamline operations, and achieve sustainable growth!
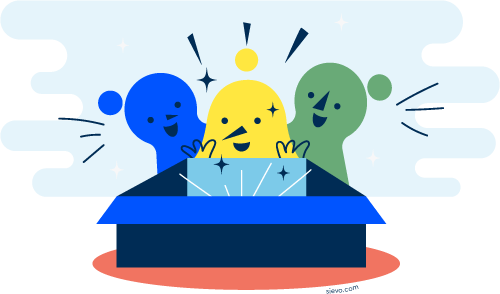
Updated: Feb 6, 2025
What is Procurement Analytics? An Introduction to Data-Driven Procurement Strategies
Data-driven procurement is transforming the way organizations manage their procurement processes by leveraging advanced analytics to unlock key business insights and drive smarter decision-making. Better, faster decision-making enables professionals to focus on strategy and relationships, rather than routine decisions.
Procurement analytics is the process of collecting and analyzing procurement data for business insights and effective decision-making. Procurement analytics involves collecting, classifying, and analyzing procurement data from multiple sources like ERPs and other systems. The data is then transformed into actionable insights, presented through visualization dashboards or business intelligence tools, to support future-proof procurement strategies.
From historical spend analysis to predictive analytics that guide future decisions, procurement analytics has evolved significantly. What once started as isolated spend analysis projects, such as spend cubes, has expanded into sophisticated dashboards, specialized software, and automation tools. Today, procurement analytics is vital for gaining a holistic view of spend, improving supplier relationships, and optimizing costs.
In this guide, we’ll explore how procurement analytics can be applied to your organization, covering essential use cases, KPIs, implementation strategies, and tips for choosing the right solutions. By mastering data-driven procurement, you can position your business for future success and operational excellence.
Benefits of Procurement Analytics
Procurement analytics is not only for procurement. It provides value to the whole organization. All other functions, from marketing to finance, can benefit from procurement data and its broad range of insights.
In procurement analytics, value comes from more timely, accurate, and actionable business insights. Also, good analytics empowers procurement's ability to measure contribution to the bottom line.
Procurement organizations can utilize analytics to describe, predict or improve business performance. It can enable effective and data-driven decision-making. Automation of repetitive tasks in procurement leaves more time and focus for strategic decision-making and relationship management.
Procurement analytics can be used in resource management, strategy planning, market research, and business development.
There is a wide array of opportunities for the organization:
-
Improving forecasting and budget management.
-
Improving risk mitigation and disruption management.
-
Gaining insights into quality management and performance development.
-
Benchmarking performance on category, unit, and country levels.
-
Discovering opportunities for consolidation, prioritization, and focus.
Types of Procurement Analysis
The field of procurement analytics has emerged from the need to understand past performance and guide future decision-making. Common types of analysis in procurement include:
-
Descriptive Analytics – where procurement data is analyzed to describe what has happened in the past.
-
Diagnostic Analytics – where procurement data is interpreted to understand why something has happened in the past.
-
Predictive Analytics – where trends and patterns in data are used to forecast future procurement performance.
-
Prescriptive Analytics – where predictive models based on procurement data aid decision-making.
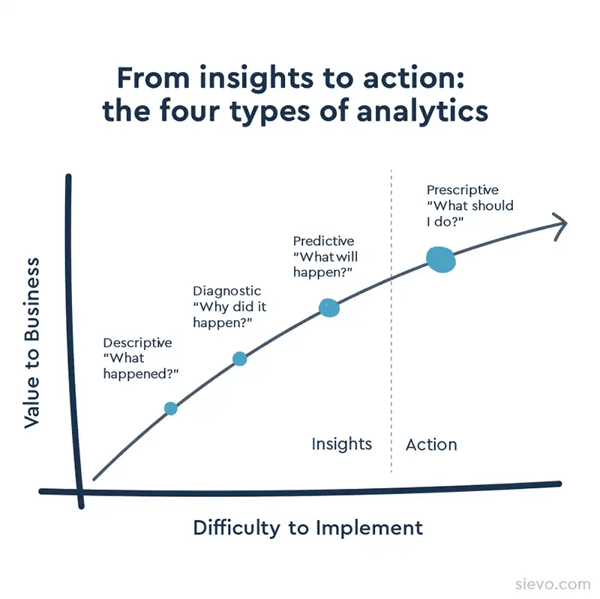
Historically, procurement analytics has focused on understanding past procurement spend and supplier performance, but increasingly focus is shifting toward automated and prescriptive decision-making.
Over time, the functionality and service models of spend analysis solutions have evolved to meet the growing needs of procurement organizations facing digital transformation.
A broad field of spend analysis solutions has emerged to serve different needs and maturity levels. There are four generations of procurement analysis solutions.
-
Generation 1 (1990 – 2000): analysis done in Microsoft Excel by consultants or business analysts with focus on past spend analysis.
-
Generation 2 (2000 – 2010): desktop spend analysis software bought under license with data hosted on-premise or within the company firewall.
-
Generation 3 (2010 – 2015): browser-based spend analytics dashboards providing business intelligence level visualizations and usability. Licensed or bought as a Software-as-a-Service.
-
Generation 4 (2015 – today): AI-powered, automated procurement analytics solutions combining many data sources. Encrypted and hosted on the cloud. Bought as Software-as-a-Service.
Different generations of solutions co-exist to meet the needs of different types of businesses. Some procurement organizations retain their Excel-based reporting, while others may continue to use their self-built or configured business intelligence solutions long into the new decade.
But we view analytics as much more than data visualization. It's about collecting, cleansing, and enriching large amounts of data to from disparate systems and external sources to create a "procurement information hub." This single source of truth can enable you to uncover insights that lead to action.
Sources of Procurement Data
Procurement organizations often face the challenge of heterogeneous data landscapes. As many businesses are formed from more than one business unit, financial processes may vary on a regional or international basis or across businesses.
Increasingly, procurement analysts are starting to leverage data from outside of their own procurement organizations, combining the most valuable aspects of internal and external data.
Internal data assets are hosted or originated within corporate applications. It is common for businesses to utilize more than one transactional database, such as enterprise planning systems (ERPs) or accounting software. Procurement analysts may also utilize data provided directly by suppliers or different business units through Excel or tap into data from the general ledger or other financial records.
External data assets are any data sources that come from outside of the company's own financial databases. These may be public systems, such as information on suppliers, commodity prices, or currencies readily available on the Internet. External data sources also include 3rd party proprietary sources, such as supplier industry codes, credit ratings, or supplier risk profiles.
In the past, independent analysts working with Excel have been limited in the ability to utilize external data sources. Cloud-based procurement analytics software and application programming interfaces (APIs) have enabled more automated, faster, and more flexible uses of procurement data.
Examples of analytics in procurement
Across different procurement organizations, there may be different applications of procurement analytics.
Some of the most common examples include:
Spend analytics: the analysis of procurement spend data from internal or external data sources.
Invoice analytics: the analysis of invoice data and payment cycles from internal or external data sources.
Purchase order analytics: the analysis of purchase order (PO) coverage, maverick spend, and PO cycle times from internal or external data sources.
Payment term analytics: analysis used to identify and act on working capital improvement opportunities.
Supplier analytics: the analysis of individual suppliers’ performance, comparison of supplier performance, analysis of supplier risk, sustainability or diversity, or analysis of supplier base.
Diversity analytics: the analysis of social responsibility and diversity in the supply base, on category and individual supplier level.
Sustainability analytics: the analysis of Environmental, Labor & Human Rights, Ethics, and Sustainable Procurement targets from your supply chain.
Supplier risk analytics: analysis of external risk factors negatively impacting your supply chain.
Contract analytics: the analysis of supplier contracts and their meta-data, such as payment terms and expiration dates.
Market benchmarking: assess risk and discover opportunities by benchmarking your purchase prices against market price development.
Savings lifecycle analytics: the analysis of savings projects and their impact on the financial bottom-line.
CO2 analytics: the analysis of scope 1, scope 2, and scope 3 emissions.
Spend forecasting: the forward-looking analysis of procurement spend data and its impact on profitability.
Use cases of procurement analytics
A common misconception is that procurement analytics only applies to spend analysis. In reality, data analytics plays a crucial role in every stage of the procurement lifecycle, from strategic sourcing to category management and procure-to-pay processes. By leveraging analytics, procurement teams can enhance decision-making, drive cost savings, and optimize performance across all functions.
Here are key reasons why procurement analytics is essential for various procurement activities and why it goes beyond just spend analysis.
Analytics in category management
When effectively used, analytics give category managers superpowers. Procurement analytics allows category managers to identify savings opportunities, segment and prioritize suppliers, identify sourcing potential, address supply risk opportunities, manage sustainability performance, develop supplier relationships and facilitate innovation.
Blog: Introduction to category management | Definitions and best practices
Analytics in strategic sourcing
The best business strategies are informed by data. In strategic sourcing, analytics helps identify the best times and areas to run sourcing events and requests for proposals. It can identify which suppliers to include in sourcing projects and provide rich information into suppliers’ quality and risk positions.
Blog: Leveraging spend analytics in strategic sourcing
Analytics in contract management
Analytics provide value across contract lifecycle management. It can alert when contracts need to be renegotiated or provide data for supplier negotiations. What’s more, analytics can identify maverick spend to help compliance and improve contract coverage.
Blog: 7 tips to reduce maverick spend in procurement
Analytics in source-to-pay (S2P) process
Procurement analytics can also provide much value in the transactional side of procurement. With analytics, you can measure purchase order cycles and improve payment terms. You can evaluate payment accuracy, discover rebate opportunities, identify mistaken payments, and reduce fraud.
Blog: How PO analytics fuel P2P performance: insights from a best-of-breed
Analytics in sustainability and corporate social responsibility (CSR)
Increasingly, companies are realizing the value of analytics in assessing sustainability and CSR and related risk within the supply chain and procurement. Analytics can uncover the environmental or social impact of procurement decisions and identify opportunities for more sustainable alternatives.
Blog: What is sustainable procurement and why is it important?
Analytics in risk management
Analytics can aide in identifying and mitigating risk within the supply chain and procurement. Analytics can unravel the complex relationships between supply, price, the environment, CSR initiatives, and risk, while identifying opportunities for mitigation.
Blog: 3 ways to tackle commodity price fluctuations and volatility
Analytics in performance measurement
Procurement analytics is classically used to identify savings realized, which is directly relevant for profit and loss (P&L) reporting for finance.
Blog: Everything you need to know about Procurement savings
Business Cases for Procurement Analytics
Procurement analytics has the potential to improve operational efficiency across the entire sourcing and supplier management lifecycle. Common business objectives for procurement analytics include:
-
Cost reduction – identification of savings opportunities and measuring savings projects' impact on the financial bottom-line.
-
Corporate responsibility - identification of opportunities for social responsibility, increased diversity and emission reduction in the supply base.
-
Risk management – identification, measurement, and mitigation of supplier or market risks within procurement operations.
-
Sourcing opportunity identification – explorations of new or more strategic ways to manage and consolidate suppliers or categories based on historic procurement data.
-
Cash flow improvement – highlight ways to improve operational cash flow, for example, through payment term optimization or currency rate optimization.
-
Business opportunities - identification of opportunities, new market areas, business insights and product/service development potential based on procurement data, external data and spend trends.
Many organizations recognize that procurement is a critical business contributor. Typically 40 – 70% of all costs are procurement-related, and these costs are often a volatile source of competitive advantage.
Effective organizations leverage data to more effectively manage supplier relationships, and growth and even bring new innovations to life.
More data has been created in the last two years than in the previous history of mankind, bringing new challenges for procurement analytics. Advancing analytical technologies accelerate the process from data to insights and unlock new opportunities.
Assessing the need for analytics
The common goal of most procurement analysis solutions is to be a ‘single source of truth’ where procurement teams can make informed decisions with data they can trust.
If the data is not reliable, you may run into a “bad data in, bad data out” situation, where costly mistakes are made based on unreliable analysis.
While most procurement organizations have similar aims for reliable data visibility, they all have unique resources and challenges to achieve the goal.
You can assess the complexity of your needs on four key dimensions:
-
Connectivity - do you need to bring together multiple sources of data from within and outside of your procurement organization?
-
Adaptability - can the solution fit into your team’s sourcing processes, culture, organization structure and workflows?
-
Transparency - do you need to see and understand how spend is organized? Can you trust the data?
-
Speed - how often do you require updated analysis and how actively is it used in your organization?
As a rule of thumb, the higher your needs for connectivity, adaptability, transparency, and speed (or CATS) the more likely you are to need procurement analytics.
If you don’t require many connections between different source systems and a high level of transparency in the analysis process, you may be able to start with a one-time analysis done in Excel or with help from a consulting partner.
If you have a complex organization or global supplier networks, or you require regularly updated data, you may benefit from dedicated procurement analytics software.
Procurement KPIs and Metrics
Procurement metrics or key performance indicators (KPIs) provide organizations with quantifiable values to measure performance and guide procurement strategies. Metrics can also be used to benchmark procurement’s performance to peers or to prove contribution to company-wide goals and targets.
One challenge with procurement metrics is that they may be used inconsistently within a procurement organization or have different meanings for key stakeholders such as finance. Here is a quick reference to some of the key metrics you can measure.
1. Spend under management
Spend under management is the total amount of spend that is actively managed by the procurement organization. This figure can include every region and category that procurement is working with, or can be divided into separate metrics that represent a specific region or category.
Spend under management is an important metric for a procurement organization because it reflects maturity and control over spend.
2. Spend vs. budget
Spend vs. budget tracks the realization of spend in procurement and compares it to the budgeted spend overall or per business unit.
Tracking realized spend against budgets is the foundation of spend management and ensures alignment with key stakeholders such as finance.
When evaluating spend vs. budget, success is not necessarily measured by a decrease in cost but by budgeting accuracy.
3. Total cost of ownership
Total cost of ownership (TCO) is the cumulative cost of all spend purchases. TCO takes into account every cost that is incurred during the procurement phase and includes all direct and indirect costs of a product or system.
It’s not limited to just the purchase price but includes transaction fees, warehousing, and other incidental costs.
TCO is a valuable metric for procurement because it provides a cost basis for the total economic value of an investment.
4. Cost Savings
Cost savings is measured by the cumulative amount of savings gained. These are then broken down by category for focused measurement. They are followed over a time period to see how cost-saving targets are met.
Cost savings could be achieved through aggregating spend across business units, taking out longer-term contracts or introducing more competition, ordering in larger quantities, implementing vendor-managed inventory systems, standardizing and rationalizing the spend, or using combinations of these different levers.
5. Cost avoidance
Cost avoidance is used to describe any actions that help a company avoid absorbing inevitable additional costs.
These costs could be due to inflation, shorter payment terms, exchange rate fluctuations, requirements for additional features or services, etc.
Negotiating with cost avoidance measures helps in keeping future costs down. Cost avoidance will not be reflected in budget or financial statements but can be used as a measure of procurement performance.
6. Average payment terms
Average payment terms measure the average time (in days) invoices are paid, calculated using every single instance of payment term information. Improving or harmonizing payment terms among and within suppliers is a key way of improving working capital.
While the improvement in working capital will not be reflected in financial statements, you can calculate savings based on proxies such as the cost of borrowing.
7. Number of suppliers
The number of suppliers informs how many distinct suppliers are being utilized in the procurement organization or in a specific category.
Reducing the number of overlapping suppliers in a category can result in efficiencies or cost savings. Increasing the number of suppliers in key categories may be advantageous to reduce supply risk.
8. Contract coverage
Contract coverage measures the amount of spend that is covered by a contract. In contrast, maverick buying highlights the possible loss of value that occurs when buying off-contract.
Increasing the amount of spend that is covered by contracts (or procurement-approved purchase orders) can result in savings while also reducing compliance risk.
9. Exchange rate exposure
Exchange rate exposure measures the changes currency fluctuations and conversions have on the overall spend.
The long-term impact of exchange rates can be measured and isolated from realized savings measurement.
10. Vendor accountability
Vendor accountability measures suppliers’ performance and how they are responsible for handling errors and claims. Examples of vendor performance measurement include defect rate, lead time, and the cumulative amount of incidents per supplier.
The goal of vendor accountability is to ensure that the overall best product or service is delivered, and the development of more strategic supplier relationships.
Once you’ve identified your key procurement metrics it’s time to develop a procurement performance dashboard. One method to utilize procurement metrics is through a balanced scorecard.
Balanced Scorecard for Procurement
A balanced scorecard is a strategic performance management framework to identify and improve various functions including financial and non-financial metrics developed by Robert S. Kaplan and Dave P. Norton.
For procurement, a balanced scorecard helps managers and teams keep track of the execution of activities and the consequences arising from these actions.
While there are some industry-level best practices, each company is likely to have its own scorecard of metrics related to its own business goals. In the balanced scorecard model the key focus areas to include are:
Financial Perspective – a small number of high-level key financial measures for the procurement organization that can be tracked and reported in a way recognized by finance.
Customer Perspective – while procurement doesn’t typically have external customers, this could include measures of customer satisfaction or responsiveness to internal stakeholders.
Learning and Growth – assessment and measures of the skills within the procurement organization and how new talents are brought in and retained in the organization.
Internal Processes – measures of the efficiency of the procurement organization. How quickly and accurately tasks are done and how they can be streamlined or automated.
Setting up metrics can be difficult, as the amount of information provided has grown over the years. Some procurement organizations highlight metrics with no actionable value.
Another mistake in performance management is having too many metrics in place. Too many trackable items can be detrimental as it leads to overanalysis. Overanalysis can lead to loss of meaningful performance, as there is a lack of an overall vision and too much focus on tracking individual metrics rather than working on what will drive improvement in the big picture.
The most important element of any procurement scorecard is clear and realistic targets. In other words, not just measuring specific metrics but developing clear goals for where each metric should be headed. It is a good practice to have ambitious goals, but unrealistic goals may demotivate the procurement organization and hurt the business.
Procurement Analytics solutions provide ready-made templates to track procurement metrics and develop procurement scorecards.
Procurement Analytics Solutions
With the increased availability of business intelligence (BI) and cloud computing technology, there has been an increase in procurement analysis solutions.
Most procurement organizations are faced with the common “build or buy” dilemma. On one hand, you can choose to build your own reporting system in either Excel or a BI platform like PowerBI or Tableau. Alternatively, you can choose to buy dedicated procurement analytics software.
Let’s go through the benefits and limitations of each option.
Business Spend Intelligence
Business Spend Intelligence (BSI) is the process of statistical procurement spend analysis to support executives in making informed business decisions. Often led by data scientists or analysts, the process involves data mining, data visualization, and reporting.
Spend Cubes are a common example of business spend intelligence, where data is presented across three axes to answer three key questions for procurement: who is buying, what is being bought, and who is bought from.
BSI can be delivered either by specialized consultants, a centralized BI function, or by analysts within the procurement organization and is typically seen as a one-off exercise conducted in Excel or as continuous reporting within a BSI tool.
Key Benefits of BI Solutions for Procurement Use Cases
Cost-Effective - Building procurement reporting with BI tools is a relatively affordable way to get an overview of procurement spend.
Alignment with Other Functions - BI tools can be integrated with wider organizational reporting systems, ensuring alignment across departments.
Key Challenges of BI Solutions for Procurement Use Cases
Outdated Data - Without real-time updates, the data analyzed may quickly become outdated, limiting the effectiveness of decision-making.
Skill Requirements - Advanced analytics skills or dedicated data scientists are often needed to handle complex data.
Maintenance and Refreshing - Regular manual work is required for data reclassification and validation, making it challenging to keep data up-to-date.
Procurement Analytics Software
Compared to self-made business spend intelligence reporting solutions, dedicated procurement analytics software provides a continuous, automated process for data analysis. These platforms go beyond just spend data, allowing organizations to monitor trends, predict future procurement needs, and optimize supplier performance.
Procurement analytics is about discovering, interpreting, and communicating meaningful insights that drive strategic procurement decisions. Dedicated procurement analytics software, such as Sievo, is specifically designed to handle procurement challenges, offering procurement-specific features, faster time to value, and the ability to incorporate multiple data sources, including supplier performance and market trends..
Example of Sievo procurement analytics software
Key Benefits of Procurement Analytics Software
Continuous Data Refresh - These platforms continuously refresh data, enabling real-time insights and timely decision-making.
Scalability - Procurement analytics software can scale with your organization’s data needs, handling vast amounts of procurement data from multiple sources without performance degradation.
Procurement-Specific Analytics - Unlike general BI tools, procurement analytics software is tailored to procurement needs, offering insights into supplier performance, category management, and contract compliance.
Faster Time to Value - Procurement analytics software typically have a faster time to value, offering pre-configured features that reduce setup time and quickly deliver actionable insights that can be turned into realized savings.
Dedicated R&D and Innovation - Providers like Sievo invest heavily in procurement-specific R&D, ensuring continuous improvement and innovation tailored to the evolving needs of procurement teams. Sievo also collaborates with industry-leading clients to co-develop new features and best practices.
Read more the 7 benefits of Procurement Analytics.
Key Challenges of Procurement Analytics Software
Total Cost of Ownership for SMEs - The advanced features and capabilities of procurement analytics software come at a higher price point compared to more limited solutions. Realized savings outweight the investment, but small and medium-sized enterprises (SMEs) with limited spend under management may take more time to break-even.
Limited Customization - While these platforms offer extensive procurement-specific features, customization may be limited compared to fully flexible BI tools. However, providers like Sievo address this with tools like Craftboards and self-service dashboards, empowering users to tailor the platform to their specific needs without having to possess analytical skills and capabilities.
Three Steps of Procurement Analytics
Procurement analytics brings visibility into spend and supplier performance and helps un-tap potential savings and opportunities. Even though spend data already exists in systems, making sense of it is often far from straightforward.
Here are the 3 general steps that take procurement data from source to screen.
1. Data Extraction
It starts with extracting the data from all possible sources and consolidating it into one central database. Once it gets extracted, data is ready to be enriched and cleansed.
Data extraction is the process that takes outdated and messy sources of information into a clean and consolidated format that can be easily understood and is ready for analysis.
In many large companies, data extraction is a challenging task that requires significant human and capital investments. On top of that, most software systems weren’t built for the intensive load an extraction will put on them, especially internal databases that need to continue serving users during the data pull.
Because of the unexpected amount of stress put on the servers, considerable performance problems can occur during the process. The amount of data to extract and the amount of maintenance required may result in longer than expected lead times for data extraction.
Pulling large amounts of data out of a myriad of sources without a standard interface can be quite a tedious task.
At Sievo, we’ve built our own automated data extractor capable of collecting data from over 100 different source systems. The Sievo Data Extractor is designed to connect and extract the most complicated and extensive procurement data from all kinds of data sources and deliver it for further analytical processing.
Here are Sievo Data Extractor’s five key strengths:
1. Speed through automation: Sievo’s Data Extractor has a pre-configured template for extraction to take the correct data, as well as the possibility for additional data fields. The tool significantly cuts down the amount of time and effort needed, including the number of people that need to be involved in the process.
2. Data security: Sievo Data Extractor represents an easy way to extract data from multiple systems with complete data security. Companies understandably want to have full control over sensitive data, and this tool guarantees a secure way of extracting data and delivering it for further processing with the possibility for customers to still keep control.
3. Quick installation: Installed to a client’s computer within minutes and compatible with practically any ERP, procurement, or finance system, Sievo Data Extractor seamlessly captures all the data needed and pushes it to the Sievo cloud.
4. Reliable monitoring: The tool allows us to monitor all processes in case of exceptions. We’ve learned through experience how to react to errors such as connections not working or only partial data sets getting extracted.
5. Data cleansing: After the transformation logic, what you’re left with is a clean, consolidated, and filtered format which then makes the rest of the process easier and faster.
Read more about Sievo Data Extractor and other Integrations
2. Data cleansing, categorization, and enrichment
Next, data has to be classified into clear and defined categories. To make spend analysis effective, precise data classification is needed, as it makes the heterogeneous spend data easier to address and manage across the organization.
This process harmonizes all purchasing transactions to a single taxonomy in order to enable customers to gain visibility on their global spending. In this step, data can also be enriched through automated translations or supplier consolidation.
Classification is about harmonizing all purchasing transactions to a single taxonomy, enabling customers to gain visibility of their global spending in order to make better sourcing decisions.
Effective procurement analytics is not possible without precise data classification. Without classification, everything is cluttered and unorganized.
Unifying heterogeneous spend data into clearly defined categories makes them easier to address and manage across the whole organization.
Complex data classification solutions are not always simple to implement, but they can be improved over time.
Sievo’s collaborative classification engine is not a black box—it is transparent. The visibility for customers makes the whole process collaborative, as the customers can freely participate and make exceptions if necessary. Once classified, the information goes standard across the organization and users can see what data characteristics the classification has been based on.
Our approach includes human + machine collaboration, where the advanced knowledge of procurement experts is complemented by our own proprietary machine learning solutions. In practice, we continuously train computers to learn and adapt the classification techniques performed by humans. The more data computers see classified, the more they learn.
Learn more about Sievo's process of classifying and enriching your data
3. Reporting and analysis
After the data has been classified, it is now ready to be analyzed. The possibilities of insights are vast. However, the most logical next step is to perform spend analysis.
Spend analysis is the practice of analyzing procurement spend to decrease costs, increase efficiency, or improve supplier relationships.
Possible types of insights can relate to:
-
Supplier segmentation across dimensions like geography, strategic relevance, spend, and delivery performance.
-
Category analysis to review spending or supplier trends over time or to identify new opportunities to improve category performance.
-
Overview of how your spend is performing compared to other points in time, such as last year, last quarter, and so on.
Spend Analysis gives you the needed spend visibility to deliver insightful analysis for accelerated opportunity identification, smarter sourcing decisions, and full control of your spending.
The principle of Source-to-Screen analytics is a smooth data journey that surfaces actionable insights. Like the iceberg metaphor, most procurement insights are hiding beneath the surface.
Evaluating Procurement Analytics software
Each procurement organization has its own way to evaluate and choose new software partners. There is no silver bullet to finding the right partner, but these common best practices can help in the process:
-
Go beyond data categorization – in the past, analytics vendor evaluations focused greatly on spend classification and master data management. These are important but today, data quality should be an enabler to more strategic insights.
-
Look for transparency – effective procurement leaders need to see the big picture but also drill down to details when needed. Analytics solutions should allow for configurable dashboards and provide data transparency all the way down to the transaction level.
-
Exploit relevant expertise – while each procurement analytics case is unique, specialist analytics vendors will have years of experience to share from similar customers or industry cases. Check for relevant customer references and look for a vendor that can guide you to the right solution.
-
Involve key stakeholders early – increasingly, procurement analytics has benefits for different stakeholders outside of procurement from corporate risk management to financial and strategic planning. If alignment with other departments like Finance, IT, and legal is important, include them early in the evaluation process.
The total cost of ownership (TCO) for an analytics solution can be divided into 3 main elements:
-
Acquisition and development cost: the costs related to the acquisition, maintenance, and development of a software solution, as well as their inherent opportunity costs.
-
User experience and functionality: the costs related to the training, onboarding, and support of users and the impact on productivity.
-
Data management and quality: the costs related to the long-term management of data, including data architecture, data quality, and data security.
All of the elements of TCO should be considered in the evaluation of the procurement analytics solution and partners.
Get a free RFP template for procurement analytics software
About Sievo
Sievo provides actionable procurement analytics based on data you can trust.
Designed for large enterprises with $1B+ in revenue, loved by global companies featuring Mars, Levi’s, and Deutsche Telekom, and praised by key analysts, we provide a future-proof solution with immediate ROI.
We empower Procurement, Finance, IT, and Sustainability teams to overcome their data chaos and capture all insights, drive bottom-line savings, improve ESG performance, and streamline budgeting and forecasting.
Sievo goes beyond world-class analytics and dashboards. We help our customers close the data-to-action gap with reliable insights, recommendations, and benchmarks by combining internal, third-party, and cross-customer data.
By processing spend data equivalent to 2% of the world's GDP annually, we enable fast, informed decision-making and deliver the industry's highest data quality with end-to-end data accountability.
Awarded and recognized by top analysts including Spend Matters, ProcureTech, and Procurement Leaders – we offer solutions for Spend Analytics, Procurement Performance Measurement, ESG Analytics, and Financial Planning and Analysis.
We call it procurement excellence - but you can call it Sievo.
Close the data to action gap with Sievo
Building your own analytics solution costs you more than you think...Learn how Sievo Procurement Analytics delivers ROI - fast.
The book for procurement data lovers.
This eBook promises a new outlook and fresh perspectives on how IT and procurement, connected by their love of data, can contribute more value to the organization—together.
An eBook that inspires data-driven procurement
Gain practical advice on data-driven leadership and utilizing procurement data ecosystems with Procurement ❤️ Data. Get your copy: