Organizations analyze procurement spend data to reduce costs, increase efficiency, and improve supplier relationships. To get the maximum value of that data, it needs to make sense.
Spend data cleansing is the process of normalizing and enriching spend data for better analytics outcomes by:
- Improving data hygiene
- Improving classification or category structure
- Harmonizing currency rates
- Translating languages across spend data, and
- Excluding irrelevant numbers from baseline calculations.
The outcome is that your data will provide better results in analytics and be more user-friendly for users across regions and use cases.
Procurement master data will always be a little messy. In larger organizations, multiple ERP systems and thousands of users can lead to data quality problems.
Most people begin a master data improvement project before implementing a spend analytics solution. But we’ll show you how data cleansing can be an on-going, automatic process.
In this blog, we’ll explain how to implement a spend cleansing and enrichment process and how automation can keep it going.
Read our full guide on Procurement analytics and the power of procurement data
Spend analysis or spend cleansing, what’s the difference?
In a very linear sense, spend cleansing happens before spend analysis, but both can happen simultaneously.
To get the most out of spend analysis, there needs to be some coherence to your procurement data. But we don’t believe in “garbage in, garbage out” as a hard rule (read Procurement❤️Data to learn why).
You can already begin spend analysis without perfect data. As you work more with the data, you can identify errors and make updates, improving the efficiency of cleansing.
Spend cleansing is a continual process since new spend data is always coming in. So, spend analysis and spend cleansing go hand-in-hand.
The same goes for data enrichment, which improves the visibility into your spend for all users.
Examples of data errors
Error-ridden spend data has side effects that impact the entire organization. It can lead to inefficient processes, decisions based on inaccurate data, loss of visibility, compliance issues, and ultimately negative customer and supplier experiences.
Let’s say you use three ERP systems across different procurement groups. Users could enter multiple supplier names, use different currencies or languages, or make small mistakes in category codes. Combining this master data for analytics will lead to much confusion.
Incorrect master data needs to be addressed before accurate analysis can be done.
Here are the most common examples of procurement data errors:
- Duplicate supplier names (e.g., PricewaterhouseCoopers, PwC, and P.W.C.)
- No standard format for dates (e.g., 1-15-23 or 15-1-23)
- No standard units of measure (e.g., litres, liters, LT, or Lt)
- General or misleading category descriptions (e.g., “Services” could mean anything from plumbing to auditing)
- Duplicated product codes (e.g., IT055 could be entered as It005, ITOO5 or similar)
- Incorrect currencies or time zones (e.g., monthly cut-off times listed in the varying time zone)
- Different document languages or broken characters
Implementing a Spend Cleansing Project: A Step-by-Step Guide
To start cleansing your procurement data, you’ll need many helping hands. One procurement person alone cannot do this. Usually, the IT department is heavily involved in managing master data.
But analytics providers like Sievo don’t need to alter your master data, only extract it for cleansing purposes.
To learn how we safely extract your spend data for analytics, read this whitepaper on the topic.
Assess the current data management practices
Start by getting a broad view of how spend data is managed. Pay particular attention to:
- How is spend data input into your system?
- How many ERP systems are in use?
- Who can change or edit spend data?
- What data policies currently exist?
- What category structures are in use?
- What spend data would most benefit from cleansing?
- What areas are irrelevant for analytics?
By getting a basic idea of the data landscape, you can identify the most critical areas first. Remember, you eat an elephant one bite at a time.
Identify areas for improvement
Once you have set the scope of data for cleansing, it’s possible to narrow down the areas of improvement. For most organizations, supplier normalization is the main challenge. Text-based fields have a high chance of error, such as category descriptions.
If your data is in generally good condition, it might make sense to focus on enrichment activities, such as translations and currency harmonization.
For international businesses with many procurement centers, having comparable data in one language and currency is necessary for large spend analysis projects.
For spend analysis, you’ll want to remove unrelated or un-addressable spend which causes noise.
Look for areas to automate
For many organizations, they will have millions of data points to consider. Machine learning and other forms of automation are incredibly efficient ways of processing large amounts of data.
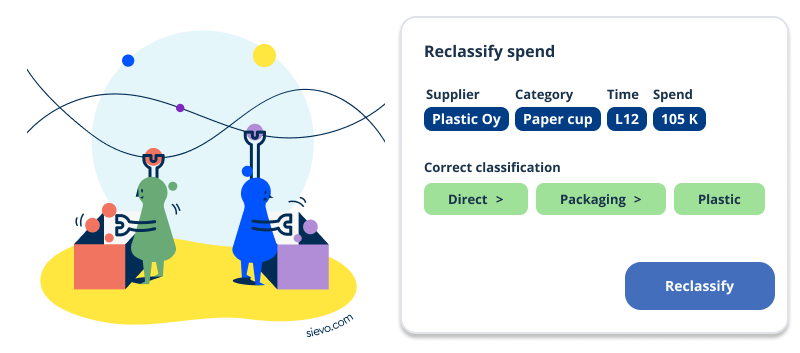
Human-machine collaboration is still necessary, but that’s even better! Training an algorithm with human oversight is much more accurate and efficient than either alone.
Here are a few examples of how automation can be used in spend cleansing and enrichment:
- Create heuristics that automatically classify new data based on a rule set.
- Supervised machine learning algorithms trained on your data can classify spend automatically.
- Unsupervised machine learning algorithms trained with unclassified data can detect patterns you can assess and learn from.
- Natural language processing (NLP) tech extracts data from unstructured text, like invoices, to process large amounts of spend data.
- Algorithms detect anomalies in your spend data and point to where human intervention is needed.
- AI scans external data sources to scrape and match new information with your spend.
The potential for AI and machine learning to automate these tasks is astonishing and powerful. However, it’s not yet foolproof. Human oversight is still a vital part of the process.
Communicate your plan and ensure buy-in
To keep your data in shape, you need to work with many stakeholders. The spend cleansing process is long and hard. So, what’s in it for them?
If you’re looking to increase the commitment of stakeholders, express that…
- Everyone in the organization has a role in improving procurement data quality
- Good data improves the accuracy and insights of analytics
- Daily users of the data have the best eye for errors
- Democratizing data increases the commitment to maintaining quality
Monitor, use, and improve
High-quality procurement data takes care and maintenance, but it shouldn’t be treated like a walled garden. A walled garden is closed off to the public.
While a few gardeners may know every plant species and their nutrient needs, they won’t be able to benefit from scale.
Continual data maintenance will be much more rewarding when more people have access. Category specialists who work daily with the data will have a better chance of catching errors.
How Sievo can help you in spend cleansing and enrichment
Procurement spend data is a rich source of insights for the whole organization.
During implementation, Sievo conducts a one-off cleansing project without changing your master data. Based on your needs, we construct automated processes that continually keep your new spend data clean.
You don’t need perfect data to gain spend visibility: our procurement expertise provides excellent results no matter the industry.
Also, you’ll gain the ability to enrich your data and make it even more insightful, with added attributes and descriptions fit for purpose. When master data is not there, our systems extract key information from other sources.
We believe in the democratization of data, which is why Sievo enables user-generated error correction with a clear audit trail.
Data cleansing is not a one-time effort. With the positive feedback loop of analytics insights, data quality improvements, and enrichment, you’ll see much greater results from your procurement data.
Contributor: Moramay Barinoff, Product Manager - Spend