AI in Procurement
The Ultimate Guide for Procurement Executives - including Definitions, Examples and Best Practices.
Want to learn how procurement pros use AI to make better decisions, faster?
CLAIM YOUR FREE AI EBOOK
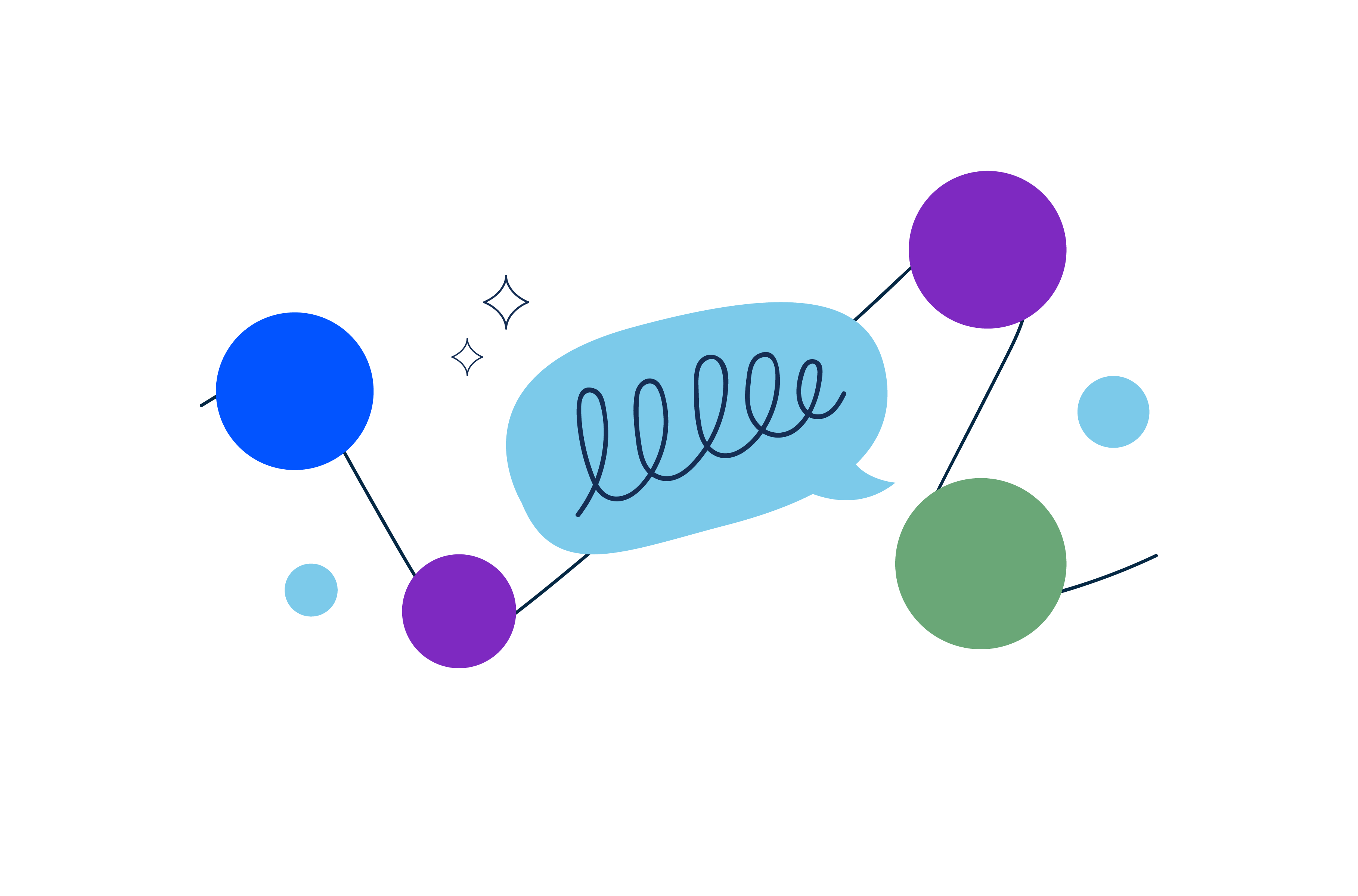
Updated: Apr 23, 2025
Often, AI is talked about as the magic wand that promises to solve all your business problems.
The talk centers around a potential future rather than a business reality.
What are the real AI opportunities in procurement? How can AI help solve some of the biggest challenges in procurement today? What are some actual use cases to help make your day-to-day job easier?
Cut through the jargon to learn what AI actually delivers for procurement professionals in 2025. This guide includes many AI definitions and examples for Procurement professionals without prior knowledge of the topic.
Click the "Claim your free AI eBook" button on the left if you want to access the full experience.
Use cases of AI in procurement
It’s 2025: You already know that LLMs like ChatGPT, Claude, or Copilot are great at taking long messages or spreadsheets and digesting them for you, but have you considered taking it to the next level by using AI’s strengths in your procurement team?
Here’s some actionable ways to use AI in procurement:
Identify More Savings Opportunities, Faster
With AI-backed spend classification and continuous data enrichment, procurement teams can confidently act on insights without the burden of manual data processing.
Classify spend with higher accuracy – Procurement teams that work with AI tend to work with clean, structured data, improving classification accuracy and coverage across all spend categories.
Expand your savings scope – AI-powered insights uncover hidden cost-saving opportunities, even in tail spend, by analyzing internal and external data sources.
Detection opportunities around the clock – AI monitors procurement data 24/7, surfacing new savings possibilities in areas like working capital, cost reduction, supplier consolidation, and sustainability initiatives.
Actionable Insights – Instead of static reports, the right AI tool can deliver ready-to-act recommendations, helping procurement teams move from analysis to execution faster.
With AI-driven insights, procurement professionals can reduce manual effort, improve decision-making, and capture more savings opportunities—all while ensuring data accuracy and relevance.
Supplier Continuity
AI enhances supply continuity by providing real-time visibility into supplier risks and market changes. By integrating internal procurement data with external sources, AI helps procurement teams proactively manage disruptions and maintain stable supplier relationships.
Comprehensive Supplier Intelligence – a decent AI tool consolidates internal, third-party, and industry-wide data to provide a 360° view of supplier performance, risk factors, and compliance metrics, ensuring procurement teams have the most accurate and up-to-date insights.
Detect risks proactively – AI can continuously monitor supplier signals, identifying potential disruptions such as financial instability, regulatory changes, or shifts in supplier ownership, allowing procurement teams to take preventive action before issues escalate.
With AI-driven supplier intelligence, procurement teams can mitigate risks, ensure supply continuity, and make informed sourcing decisions—all while maintaining a proactive approach to supplier management.
Better supply chain intelligence
AI enhances supply chain visibility by harmonizing procurement data and integrating diverse information sources, enabling procurement teams to make more informed, strategic decisions.
Accurate, Harmonized Procurement Data – AI can deduplicate and standardize material data, ensuring procurement teams can compare prices, consolidate ERP records, and refine spend classification across global and local operations.
Comprehensive Supplier Insights – AI processes structured and unstructured data, providing a 360° view of suppliers, including risk factors, sustainability commitments, and payment term recommendations.
Integrated Sustainability and Risk Data – AI enriches procurement data with third-party and industry-wide insights, helping teams evaluate supplier diversity, ESG performance, and compliance risks in a single, actionable view.
With AI-powered supply chain intelligence, procurement professionals can improve data accuracy, enhance supplier evaluations, and ensure alignment with sustainability and compliance goals.
Types of AI in Procurement
From a Procurement perspective, any software solutions that include self-learning, and smart algorithms can be considered AI. You can see examples in later chapters of this guide and refer to these common definitions
-
Artificial Intelligence (AI): any algorithms exhibiting any behavior considered ‘smart.’
-
Machine Learning (ML): algorithms that detect patterns and use them for prediction or decision making.
-
Natural Language Processing (NLP): algorithms that can interpret, transform, and generate human language.
-
[NEW!] Agentic AI: Agentic AI is a more autonomous form of artificial intelligence designed to analyze data holistically, offer proactive recommendations, and even automate decision-making when appropriate. Read more about how Agentic AI is closing the Data-to-Action gap in procurement.
All forms of AI involve algorithms – sets of rules specifying how to solve a specific problem. Algorithms can be calculated by anyone gifted in math, but they also form the basis of most computer software. The work of algorithms in software is not visible to the human eye, but they can be programmed and re-programmed by experts to solve problems deemed important within software environments.
How AI Can Help in Procurement
AI thrives at solving complex problems involving large amounts of data but clearly defined measures of success. A study by Harvard Business Review and Deloitte explored the key areas where business executives expect to see the most success with AI. While each organization has its own challenges and opportunities, these can be the areas where AI can bring value to Procurement.
Key areas AI can support Procurement:
-
Make better decisions – artificial intelligence can provide timely analytics and data-driven insights to make better sourcing decisions.
-
Identify new opportunities – shifting through vast amounts of data, AI can uncover new savings or revenue opportunities.
-
Improve operations – artificial intelligence has the potential to streamline or align internal business operations, even in large organizations with many business units or geographic locations.
-
Automate manual tasks – AI can automate many time-consuming tasks, such as monthly processes, or Procurement performance reporting.
-
Free up time – by taking care of more routine tasks, AI can free up Procurement resources for more creative or strategic tasks like key supplier relationship management.
-
Capture or apply scarce knowledge – artificial intelligence can help Procurement organizations capture relevant new sources of data, for example, from external data sources like the Internet.
-
Identify new suppliers or markets – with access to vast amounts of external data, AI can help identify new suppliers or even new markets to enter.
-
Optimize supplier relationships – AI has the potential to make supplier relationship management more data-informed.
Automation in Procurement
Procurement automation means automating your procurement processes to maximize efficiency and reduce cycle time. Automation frees employees from repetitive, manual, and time-intensive tasks and speeds up the entire process.
Automate your procurement cycle in 6 steps:
-
Map your current procurement process
-
Audit the current procurement process
-
Identify key areas for automation (labor-intensive, repetitive bottlenecks)
-
Choose the analytics solution that supports automation
-
Build automation workflows and approval points
-
Measure automation success and improve
Examples of Procurement AI
While the adoption of AI in business applications is at the beginning of its journey, there are an increasing amount of examples of AI being used within Procurement functions. Machine-learning algorithms are widely used in spend analysis to improve and speed up a number of processes, including automatic spend classification and vendor matching, and much more on the horizon.
Machine learning spend classification
Examples of spend classification techniques include:
-
Supervised Learning in Spend Classification – when humans train algorithms to detect patterns in spend, removing dull work of repetitive new spend classification.
-
Unsupervised Learning in Vendor Matching – when algorithms are programmed to detect new and interesting patterns in vendor relationships without intervention or support from humans. For example, if you have DHL, DHL Freight, Deutschland DHL, and DHL Express in your data, the machine learning algorithms are easily able to consolidate these together as DHL for increased visibility and data coherence.
-
Classification Reinforcement Learning – where spend classification actions taken by algorithms are reviewed by humans and rewarded or punished depending on the consequences.
However, when building a business case for AI, keep in mind that 100% automation isn’t always realistic. Quite often 80% of a process (such as spend classification) can be automated, but the last 20% may require a human touch. Use this 80/20 rule when considering how much time an AI-driven process will take and how it will improve current timelines.
Capturing Supplier or Market Data
Use techniques such as natural language processing to look for and capture data on suppliers or specific markets. For example, tracking social media channels for signals about suppliers' risk positions. Better predictions such as price predictions, maintenance needs, and stock market forecasting can be improved by AI.
AI can be utilized to take advantage of new sources of data. So-called “external” data sources can include market indices, company credit ratings or publicly available information about suppliers. AI-powered methodologies can sift through immense amounts of external data to identify opportunities and provide benchmarks and recommendations for improving performance.
Let’s take, for example, the task of benchmarking your performance to those of others. Say, at the moment, you are mainly using internal data as well as a static historical data set to benchmark your performance. This way you may get a fairly accurate picture but are still missing out on some key observations. A whole new level of insight comes into play when external data, such as market reports and stock prices, enter the field.
Anomaly Detection
Automated notifications on anomalies, new opportunities, and recommended activities directly in your procurement dashboards may sound like a dream but could be a reality very soon. As AI processes an ever-increasing amount of data, it is able to stay up-to-date on the latest developments and changes in the operating environment.
This will enable all anomalies and changes to be noted instantly and more accurately. AI will be able to immediately notify the team if something abnormal has occurred and can give instant suggestions on what could be done.
It can also be able to showcase possible simulations for different scenarios and new opportunities utilizing the data it has access to. The end result is that human procurement practitioners will be more aware of what is happening and will be able to take action sooner.
In addition, users can trust that the recommendations AI makes are based upon real facts rather than human hypotheses or guesswork. This gives the procurement leaders the confidence that their decisions are based upon real data, which removes uncertainty and leads to better decisions.
GenAI for procurement
Generative AI is a broad class of AI characterized by the capability of generative text, images, or other media by learning patterns and structures based on input data. Large Language Models (LLM) like OpenAI’s GPT are one of the most exciting developments in recent years.
As GenAI continually progresses, what seemed futuristic yesterday is today's reality. Procurement organizations (who deal with mountains of data) have begin experimenting with GenAI use cases.
Wondering how Sievo integrates GenAI into its solutions? See here!🤖
Synthesizing unstructured data
Training a model with text-based records of past supplier interactions and documents to generate automated “flag” protocols, summarize discussions, and more.
Capturing external data and making it useable
The possibilities here are endless, but a simple application might supplier-specific news based on articles and other internet media online, summarized and delivered to you.
Generating briefs and text-based documents
With a click of a button, you could be able to generate a summary of a supplier relationship or generate SOWs, RFPs, and POs.
Data classification and mapping
LLMs are perfectly fit to increase procurement’s effectiveness in data processing, such as in classification, CO2 mapping, supplier normalization, and data engineering/ mapping data.
Automated vendor communication
Needles to say, these chat tools are powerful enough to handle complex and human-like conversations. GPT can generate human-like communications to streamline the process of requesting and receiving information from suppliers, such as pricing, lead times, and product specifications.
Risk Mitigation and Compliance
LLMs can analyze contracts, identify potential risks, and suggest mitigation strategies. Comparing contract data to regulatory information can help procurement monitor regulatory changes and alert teams to any updates that may impact their supply chain.
Read more about ChatGPT for Procurement and our view on the hype
Procurement AI Software
According to Deloitte High Performing Chief Procurement Officers 18x more likely to have fully deployed AI/cognitive capabilities. Here are 7 common areas where AI can be used across the procurement cycle.
Contract Management Software
AI has many potential use-cases in contract management. One very concrete example is natural language processing, which enables software like Docusign Insights to automatically scan and interpret lengthy and verbose legal documents for potential savings opportunities.
Supplier Risk Management
Artificial intelligence can be used to monitor and identify potential risk positions across the supply chain. For example, in RiskMethods RiskIntelligence, big data methodologies are used to screen millions of different data sources and provide alerts in supply chain risk management software.
AI in Purchasing Software
Artificial intelligence can be leveraged to automatically review and approve purchase orders. For example, in Tradeshift’s platform, a chatbot called Ada can be used to check the status of purchases or automatically approve virtual card payments.
Accounts Payable Automation
Increasingly, machine learning is being used in accounts payable automation. Stampli is one example of AP automation software that uses machine learning to speed up payment workflows and detect fraud.
AI Spend Analysis Software
In procurement spend analysis machine learning algorithms are widely used to improve and speed up a number of processes, including automatic spend classification and vendor matching. We’ll go through this example in more detail in a separate chapter of this guide.
New Supplier Identification
Big data techniques enable new ways to identify, manage and utilize supplier data across public and private databases. Platforms such as Tealbook use machine learning to enhance supplier discovery based on information gained, cleansed, and enriched from the Internet.
AI in Strategic Sourcing
Artificial intelligence can also be used to manage and automate sourcing events. Keelvar‘s sourcing automation software uses machine learning for the recognition of bid sheets and has specialized category-specific eSourcing bots such as raw materials and maintenance/repair.
Supervised learning with Procurement Data
Most uses of artificial intelligence in business contexts require some human supervision. When you see implementations of AI in procurement, they are likely to involve supervised learning. In these cases, procurement experts are included to actively train machines to perform a specific task.
Here is how you could train AI with procurement data:
-
As a first step, a set of training data is given to an AI algorithm with a specific challenge. For example, it could be asked to observe how 100,000 invoices have been classified into different categories of spend.
-
With a clear goal and some training data in mind, you could then begin to give the AI algorithm unclassified Procurement data to classify based on the logic it has observed in the training data.
-
In cases where AI has high confidence it can classify data correctly, the data would be automatically categorized without human input.
-
In cases where AI does not have high confidence, the classification decisions would be reviewed by Procurement experts. This process is sometimes called “human annotation.”
-
-
With feedback from human reviews, Procurement Data would be both classified and utilized to actively teach the AI algorithm to classify future data.
-
Over time, the confidence of the AI would improve to automatically classify more data, while also increasing the quality of data classification based on the human input.
When you consider this training process from the point of view of a Procurement organization, consider what are the tasks at hand where you would have enough training data, a need to consistently process unclassified data, and a concrete output that adds business value.
Use of Machine Learning in Procurement
Machine learning in procurement is the application of self-learning automated statistics to solve specific challenges or improve operational efficiency. With machine learning (ML), procurement can deliver the highest quality in relation to volume and bottom-line impact.
Machine learning is the subset of AI with the most immediate applications within procurement. It is a natural successor to robotic process automation (RPA) in the evolution of automated or autonomous procurement processes. While RPA is considered automated statistics, it lacks the ability to learn and improve over time.
Machine learning is also one of the most misunderstood aspects of AI in procurement organizations. Some enthusiasts consider any examples of advanced statistical methods to be ML, while many software vendors portray images of human-like machines. It’s time to dispel some myths by reviewing the core types of machine learning.
4 Machine Learning applications in procurement
Different types of machine learning are used within procurement processes. Each machine learning type requires a varying degree of human intervention. The four types of machine learning include:
-
Supervised Learning: an algorithm is taught the patterns using past data, and then detects them automatically in new data. Supervision comes in the form of correct answers that humans provide to train the algorithm to seek out patterns in data. Commonly used within spend analysis in areas such as spend classification.
-
Unsupervised Learning: the algorithm is programmed to detect new and interesting patterns in completely new data. Without supervision, the algorithm is not expected to surface specific correct answers instead it looks for logical patterns within raw data. Rarely used within critical procurement functions.
-
Reinforcement Learning: the algorithm decides how to act in certain situations, and the behavior is rewarded or punished depending on the consequences. Largely theoretical in the procurement context.
-
Deep Learning: an advanced class of machine learning inspired by the human brain where artificial neural networks progressively improve their ability to perform a task. Emerging opportunity in procurement functions.
Machine Learning for Spend Analysis
Machine learning is already widely used across data-intensive processes such as procurement analytics. Let’s deep-dive into how machine learning is used in spend analytics and spend classification, and specifically to tackle the spend classification challenge.
Spend analysis is the process of identifying, collecting, cleansing, classifying, enriching, and analyzing your organization's spend data.
Spend Classification problems and challenges
Classifying spend into procurement categories is the key challenge in procurement spend analytics. Spend classification is one of the first applications of artificial intelligence in procurement. AI is broadly utilized today in automating the classification process.
Challenge 1 - Spend categorization
Procurement organizations face the need to categorize millions of unique transactions into procurement categories. Transaction data is coming from invoices, purchase orders or other data sources. Procurement organizations with good intentions create complex hierarchies of categories and sub-categories but often find it difficult to maintain a high data quality or efficiency in categorizing new data.
Challenge 2 - Need for hyper-relevant data
In the past, it was common that procurement spend was analyzed once a year or each quarter. Automation of processes enables shorter spend analysis cycle time. Today, high-performing Procurement organizations rely on nearly real-time data updates to meet the changing business needs.
Challenge 3 - The amount of data is growing
Another key challenge is that the amount of data available is growing across different source systems. There is an increasing challenge to connect heterogeneous sources of data. Procurement organizations may need to bring together spend data from across a number of enterprise planning systems (ERPs), purchase-to-pay solutions or other finance-related software. Each source system may contain only some relevant data points, and there is a need to connect disparate spend data into one hierarchy.
Spend classification example: IT hardware
For example, a new computer may be labeled as IT equipment in the general ledger, while the invoice line description provides additional detail distinguishing it as a desktop computer. The purchase order for this item may even have a different description, referring to a vendor or maker-specific data points. While all these data sources refer to the same item, it requires intelligence to make a correct classification.
AI in Procurement Spend Classification
While different aspects of AI have the potential to solve or reduce the spend classification challenge, most software solutions today involve some form of supervised machine learning. Let’s go through a concrete example of machine learning-powered spend classification. Sievo’s spend analytics has a number of concrete ML use cases:
-
As a starting point, machine learning algorithms can automatically classify new spend data into procurement taxonomies.
-
The software can also provide suggestions for category experts through a classification tool (see screenshot below).
-
In addition, the machine learning-powered AI-classifier can give a confidence level from 0 to 1 for each classification suggestion. Low confidence is closer to zero while a larger number depicts higher confidence.
-
Machine learning can also go beyond new spend classification. It can spot errors made in previous rule-based classifications by human category experts.
-
A human category expert can review or validate AI-classified data and provide valuable training input for future classifications.
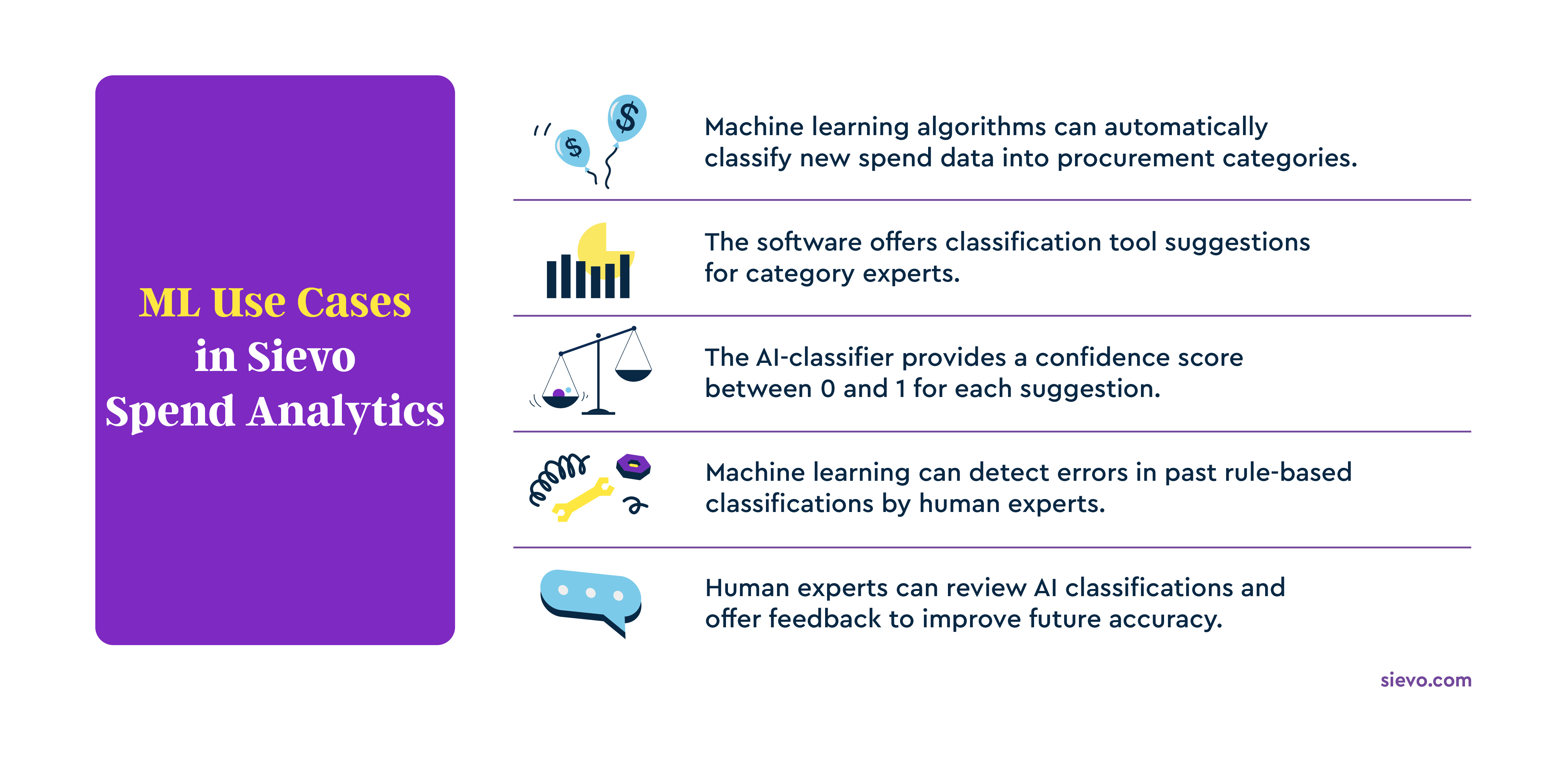
Natural Language Processing (NLP) in Procurement
Natural language processing (NLP) is the branch of artificial intelligence focused on understanding, interpreting, and manipulating human language. For procurement, NLP can uncover insights from existing data or enable new ways to streamline time-consuming processes. Let’s go through some concrete examples. Data contained in legal contracts and documents can be parsed (interpreted) by AI for procurement of relevant information.
NLP in Contract Management
Legal contracts contain a lot of valuable information for procurement, for example, on termination dates, payment terms and re-negotiation rights. Historically, this information has not been readily accessible to procurement teams because contracts have been written in contractual terms and stored offline or on shared online folders where the data contained in contracts has not been readily available. Natural language processing has enabled procurement to mine contracts for valuable data through a method called text parsing. Contract management software can utilize parsing algorithms to efficiently scan and interpret even large amounts of contracts for critical information. Taking this even further, optical character recognition (OCR) is an AI-enabled approach that interprets and identifies text automatically from any images, including photos of previously un-digitized scanned contracts.
Word Embedding in Invoice Descriptions
AI software and algorithms are much better at interpreting numbers than human language. A simple analogy is that computers think in terms of binary systems (ones and zeroes) while humans think in terms of words. Word embedding is a form of NLP where words and phrases in vocabulary are mapped in similarity and relation to other words. Word embedding can be useful for procurement in the analysis of text fields in purchase orders, identifying groups of purchased items that come within a similar category or sub-category.
Natural Language Generation in Chat Bots
Chatbots and personal assistants are among the most talked-about applications of AI that rely on natural language generation (NLG). These take NLP further by first interpreting human input and then giving a response in a written narrative. Voice-based assistants like Siri or Alexa are already widely used in consumer applications, but NLG is currently limited within procurement to pre-configured chatbots or virtual assistants that automate very limited tasks.
Cognitive Procurement Explained
Cognitive procurement is one of the most talked-about buzzwords. With new technologies and AI opportunities come new terms and definitions.
What is Cognitive Procurement?
Cognitive procurement is the process where self-learning AI techniques are used to mimic human intelligence. These self-learning AI techniques include automated data mining, machine learning, pattern recognition, and NLP. The phrase "cognitive procurement" originates from an emerging field of advanced computer science, “cognitive computing.”
What is Cognitive Computing?
Cognitive Computing (CC) refers to any hardware or software that mimics the functioning of the human brain and helps improve decision-making. It models how the human brain senses, reasons, and response to stimulation to solve specific tasks or challenges.
What is Cognitive Analytics?
One way in which cognitive computing can relate to Procurement is through cognitive analytics (CA). CA is a new approach to generating insights from large amounts of structured or unstructured data by mimicking a human brain’s ability to interpret patterns and draw conclusions from data. While many procurement analytics challenges may be solved by cognitive analytics, not every AI-assisted analytics solution involves cognitive solutions.
What is Cognitive Sourcing?
Another way that cognitive computing can support Procurement is by assisting sourcing processes. Cognitive sourcing can help buyers and Procurement teams to identify new opportunities or automate non-strategic sourcing activities. Sourcing assistants such as chatbots can be considered examples of cognitive sourcing.
Challenges with Cognitive Procurement
The field of cognitive computing is relatively new, so it should be approached with caution. There is not yet a broad consensus on core definitions and what constitutes “cognitive” processes in a business context. At the same time, many software solutions are being offered with the promise of embedded human-like intelligence. While technology is developing fast, it is recommended to validate assumptions within cognitive procurement with internal or external information systems experts.
AI Best Practices in Procurement
Start with boring problems
To start with AI, don’t look for miraculous new solutions to change the way you run your Procurement operations. Don’t think of AI as magical new technology. Instead, think of AI from the business process point of view. Consider the challenging but boring business operations that already take time and resources to manage. The most immediate value of AI will not come from new applications, but from embedding technology into existing processes – for example, improving your existing spend analysis or contract management processes.
Capture all possible procurement data
Another general rule of thumb is to capture as much data relevant to Procurement as you can before you know how to use it. Don’t wait for your data quality to be perfect. Instead, assume that AI technologies can help you interpret and improve historic data quality over time. The key is to collect more data for AI to interpret. The more data you give AI to train on, the better results you’re likely to get.
Give AI clear procurement challenges
In its current state, AI and machine learning are very good at narrow use-cases. You can utilize machine learning to categorize Procurement costs based on invoice line items, but you’re not likely to have AI take over complex supplier negotiations. Evaluate which routine tasks require a lot of your procurement team’s time but have clear outcomes on performance.
Be open to experimenting
While AI has the potential to improve Procurement performance over time, there are still many uncertainties. Be open to experimenting. Consider giving emerging AI technology specialists challenges and training samples of your data. Allow for mistakes and learning, and focus on the expected business benefits. Recognize also that technology is developing at a fast pace, so failed experiments of tomorrow can be possible with the new AI methods of today.
Enable Human + Machine Collaboration
Finally, remember that all implementations of AI in Procurement will require active guidance and support from Procurement experts. Plan for human and machine collaboration, where your Procurement team’s expertise is augmented, not replaced, by artificial intelligence. Be the champion of change to make the best use of both human and machine intelligence.
Future of Procurement
No one can truly know where we will be in the next 10-20 years, but some conclusions can be brought forward in terms of what will be possible in the near future for AI and procurement. There is quite a strong consensus amongst the analyst community that the applications that are already in use will develop in the future.
According to McKinsey, tasks like payment and invoice processing, placing and receiving orders, and managing demand for purchases are fairly easy to automate. In fact, many of them already are. However, tasks such as vendor selection and negotiation as well as vendor management are more difficult to automate. Although we will see a lot more automation of simple tasks, don’t expect to automate all your tasks very soon.
It’s impossible to say with certainty where Procurement AI will take us in the end, but we made some predictions on what level of maturity AI could possibly achieve:
Total process automation: No human involvement will be needed in operational procurement such as routine processes, approvals, compliance, and reporting.
Automated value creation: Machines may be able to make decisions and take action on savings and value generation opportunities without human input.
Full spend transparency: All procurement-related spend could be leveraged and available whenever key stakeholders need it – with no errors or faults.
Agile supplier ecosystems: Managing strategic supplier relationships will take a completely new dimension, as data flows freely between partner systems.
AI will provide recommendations and take actions based on data across the ecosystem, not just based on the data of a certain player. These are hypothetical scenarios, but they could be the possible culmination of the current AI applications.
The future of procurement depends on its ability to deliver measurable business value. Transformation of procurement aims to maximize procurement ROI (return on investment) in terms of:
-
cost savings,
-
efficiency,
-
collaboration,
-
innovation,
-
sustainability,
-
and financial success.
The procurement ROI is measured by comparing the procurement function’s costs with the cost savings it generates. These cost savings can further enable investments, research & development, improved customer experience, sales enablement, sustainable offering, and more. This is where AI can increase the impact of procurement.
FAQ
Popular questions about artificial intelligence among procurement professionals include the following.
Should organizations change their processes to accommodate AI? Should AI work with existing processes?
By and large, AI can be applied to existing processes, so it’s not like you need to fix something before you can start to capture the benefits of AI. At the same time if there’s one piece of advice on how to prepare yourself to leverage AI, now and in three to five years’ time, try to capture as much data as you can, even if you can’t process all of that data right now. When you have mass data amounts accessible you may be able to benefit from them in surprising ways in the future. To prepare to benefit from AI capture data even though you don’t know how to use it
Is AI in procurement only for larger organizations? Is there a business case for smaller procurement organizations?
Think of machine learning as just a new form of software. Software today is being used widely across all types of businesses, big and small. If you are Deutsche Telekom or Walmart you may have very different software needs than if you are a small business. If you are an SME you probably wouldn’t go about building your own machine learning application just like you probably wouldn’t code your own custom software. You would just look for the right software providers to suit your needs and these are already starting to emerge.
Is there a Forrester Wave or Gartner’s Magic Quadrant for AI in Procurement?
There is currently no resource like this because the applications of AI are so broad. In procurement applications, most of the value of AI today is embedded into existing solutions, for example, existing contract management or procurement analytics solutions. AI is more of an enabler than an application itself, although many people seem to be looking for a new concrete application.
What does AI governance look like? How do you govern machine learning?
There are three kinds of human-machine collaboration models when it comes to AI.
The first is called “human in the loop”, which basically means that whatever the machine does there’s always a human to see the result and approve, disapprove, and so forth. This is a good approach if those decisions are supercritical or if there’s a rather small number of those decisions to be made and they are high value.
Then there’s the “human on the loop” which means that the machine does the stuff on its own, but there’s a human supervising the process, which really works well when you have a routine task.
The third option is “human out-of-loop” which lets the machine learning run and go rogue and there isn’t a way for you to intervene. An example of human out-of-loop is financial markets and high-frequency trading. In this case, speed is of the essence, which requires you to let the machine do the decisions because humans are just too slow to intervene.
Whenever you apply machine learning you should think of what sort of model you want to apply. “Human-in-the-loop”, I will change or approve each decision, “human on-the-loop”, human actively supervises, or “human out-of-loop, I let the machine run and trust it to do its job.
Is machine learning biased, if it relies on humans for training?
AI is only as good or bad as the behavior we show the AI in our training data. For some applications, you can really take out the human bias. For example, you can train a machine to objectively distinguish between benign and malign skin conditions like cancers. Then there are other applications where it’s super hard to stay unbiased. More and more AI is used to process employee candidates, and go through the CVs and applications. It’s very difficult to remove human bias from this process. If recruiters or AI developers have a bias based on gender, ethnicity, or something like that, all those biases could be coded into the process. To try and stay unbiased you need more than one source for your training data or alternatively a rigorous vetting process for bias.
About Sievo
Sievo provides actionable procurement analytics based on data you can trust.
Designed for large enterprises with $1B+ in revenue, loved by global companies featuring Mars, Levi’s, and Deutsche Telekom, and praised by key analysts, we provide a future-proof solution with immediate ROI.
We empower Procurement, Finance, IT, and Sustainability teams to overcome their data chaos and capture all insights, drive bottom-line savings, improve ESG performance, and streamline budgeting and forecasting.
Sievo goes beyond world-class analytics and dashboards. We help our customers close the data-to-action gap with reliable insights, recommendations, and benchmarks by combining internal, third-party, and cross-customer data.
By processing spend data equivalent to 2% of the world's GDP annually, we enable fast, informed decision-making and deliver the industry's highest data quality with end-to-end data accountability.
Awarded and recognized by top analysts including Spend Matters, ProcureTech, and Procurement Leaders – we offer solutions for Spend Analytics, Procurement Performance Measurement, ESG Analytics, and Financial Planning and Analysis.
We call it procurement excellence - but you can call it Sievo.
Ready to see Procurement AI in action?
Not using AI in procurement? You're missing out on strategic cost reduction!
- capture more savings with less effort
- accelerate your decision-making
- achieve 98% classification coverage.
Take your copy of our full-length eBook!
Cut through the hype and get an executive view of the real opportunity artificial intelligence gives to procurement.